Peter Majors
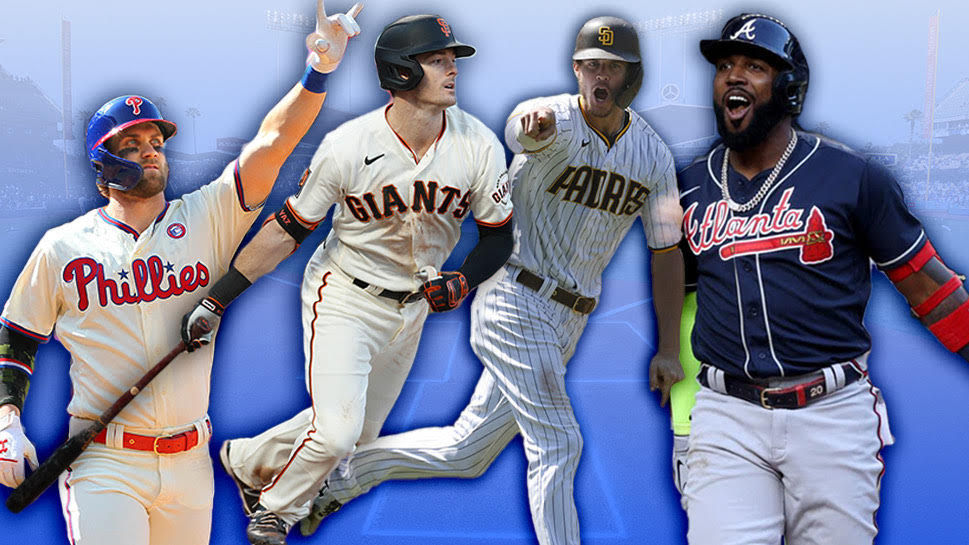
Introduction
We can all agree that this past year was one of the wildest in recent history - and as I’m sure you are aware - professional sports were not immune from all the chaos. In mid-March, Major League Baseball’s Spring Training was cut short due to concerns over Covid-19. In the months that followed, labor disputes between the league and its players association drastically delayed the 2020 season. In the end, Major League Baseball's sacred 162 game season was cut short to a measly 60 games.
Upon the league’s announcement of this shortened season, some fans grew wary about the legitimacy of the statistics produced during the upcoming season. They were concerned that a limited sample size would magnify the performances of players and teams who played above or below their actual skill level.
Questions like “Could we see the first hitter since Ted Williams in 1941 to hit .400?” and “Could we see a starting pitcher post an ERA lower than Bob Gibson’s legendary 1968 mark of 1.12?” circulated in the minds of fans. While the answer to both of these questions turned out to be “No,” this past season was still pretty wacky in its own regard.
In terms of pitching, the 2020 season featured three qualified pitchers (Shane Bieber, Trevor Bauer, and Yu Darvish), with an Earned Run Average Minus (ERA-) of 45 or lower. For context, the last time that happened was in 1884 - the same year the league decided that pitchers should be allowed to throw overhand!
In terms of hitting, the 2020 season featured three qualified batters (Juan Soto, Freddie Freeman, and Marcell Ozuna) with a Weighted Runs Created Plus (wRC+) of 179 or more. For context, the last time that happened was in 2002, during the tail end of the steroid era. If we were to ignore that period in baseball history, the last time it happened was back in 1961 - when the legendary Mickey Mantle was still in his prime.
While I'm sure these anecdotes do an effective job of communicating just how wild this past year's season was, it's time we look at the 2020 season through a bit more of a scientific lens.
More specifically, let's try to answer two important questions: "Was Major League Baseball's 2020 season, in terms of the variance of its offensive metrics, statistically distinct from those of years past?" and "Which statistics from this past season, if any, had variances similar to that of a full-length season?"
Background on Reliability
However, before I attempt to answer each of these two questions, I would be remiss if I did not offer a brief background on the reliability of statistics in baseball. According to this article, written by Jonah Pemstein of Fangraphs,
“ … reliability is a coefficient between 0 and 1 that gives a sense of the consistency of a statistic. A higher reliability means that there’s less uncertainty in the measurement. Reliability will go up with a larger sample size, so the reliability for strikeout rate after 100 plate appearances is going to be much lower than the reliability for strikeout rate at 600. Reliability also changes depending on which stat is being measured”.
One can calculate the reliability of a statistic using a myriad of methods. However, some of the most popular include Kuder-Richardson, Cronbach’s Alpha, and Split-Half Correlation. For an overview of a few of these methods, I would refer you to this article from Fangraphs - and for a great analysis that wrangles with the complications of performing an analysis of reliability, I would refer you to this article, also from Fangraphs. In recent years, each of the above methods has been used to try to identify which statistics in baseball are the most reliable.
Each time one of these methods has been employed, they achieved a slightly different set of results. However, there was one commonality that did arise between every one of these studies: statistics that individual players are more in control of are always more reliable than those they are less in control of, and correlate better with future performance. While this discovery is not entirely surprising, it is important to remain aware of as we move forward in this analysis.
In the years leading up to 2020, new research on the reliability of baseball statistics began to stall. A lack of new metrics and extensive prior coverage of the subject made it difficult to explore new ground. However, this past year’s season threw a wrench into many of the sabermetric communities’ prior analyses and opened the subject up for discussion once again.
As I mentioned in the introduction, one of the issues with this past year’s season was that players could not accumulate the same amount of plate appearances and innings that they would have if the season had not been shortened. This resulted in drastically smaller sample sizes, which lead to a major increase in the variance of statistics across the league. Despite an abundance of research on reliability in prior years - no article, at least to my knowledge - has attempted to measure the exact impact the 60 game season had on the viability of statistics. This is what I attempted to accomplish in this analysis, so I hope you find it worthwhile.
Analysis
For this analysis, I chose to employ an F-Test for Equality of Two Variances, a type of hypothesis test used to determine if two variances can be considered statistically equivalent to one another. I chose this method for its scalability, flexibility, and relative simplicity.
The statistics I considered in this analysis were:
Batting Average (BA)
Slugging Percentage (SLG)
On-base Percentage (OBP)
Expected Batting Average (xBA)
Expected Slugging Percentage (xSLG)
Expected On-base Percentage (xOBP)
Weighted On-base Average (wOBA)
Weighted On-base Average On Contact (wOBAcon)
Expected Weighted On-Base Average On Contact (xwOBAcon).
While I only included batting statistics in this analysis, this method can easily be adjusted for pitching statistics. I started by gathering all of the above statistics for each qualified batter from the 2017, 2018, 2019, and 2020 seasons. In 2020, to become a qualified batter, you had to accumulate a minimum of 186 plate appearances. Meanwhile, in 2017, 2018, and 2019, that same minimum threshold was set at 502 plate appearances.
After collecting my data, I calculated the variances for each of these statistics by season, which is represented visually by the graph below.
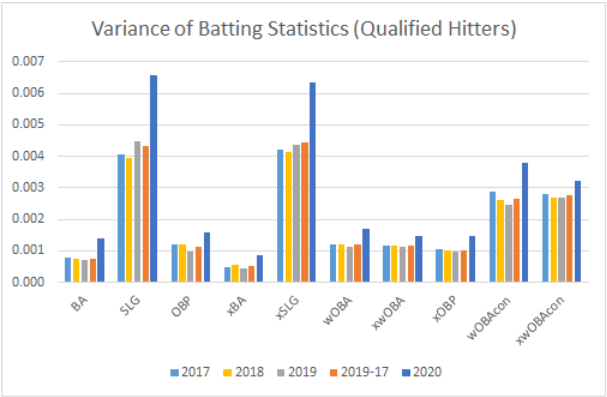
As you can see, 2020 offered far more variance than any other season in recent history. And, as the graph makes abundantly clear, these increases in variability were not felt equally among the statistics in question. While looking at this graph, it is important to remember that although statistics such as SLG and xSLG possessed the greatest increase in variance from prior seasons, their surges in variance should only be interpreted relative to their variance in prior seasons.
The way to account for this problem is by calculating Test Statistics (F*) for each variance comparison. My Test Statistics (F*) in this scenario were a ratio of variances where the larger of the two values served as the numerator for each season-to-season(s) comparison.
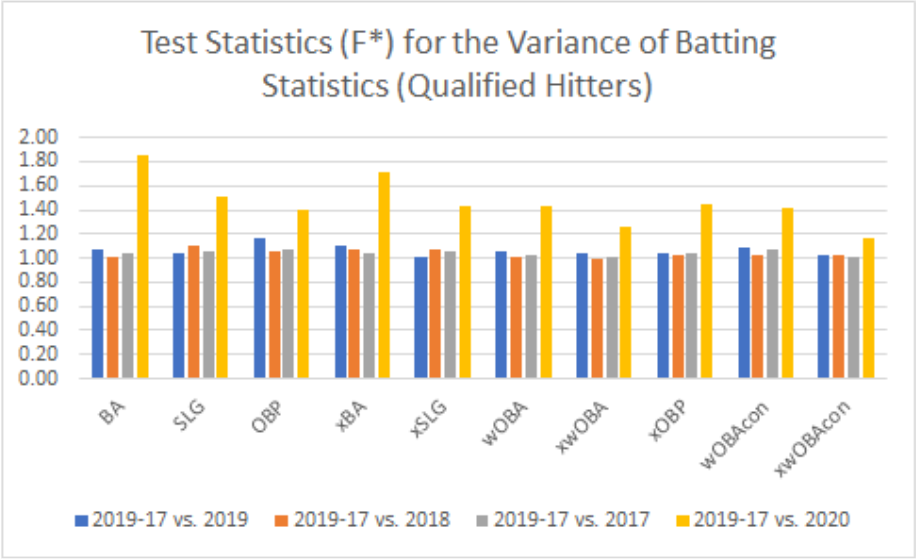
As you can see, when the 2020 season was paired up with the pool of 2019-2017 seasons, BA possessed the greatest uptick in variance among qualified batters, meaning it was the least reliable metric for assessing batter performance this past season.
After calculating my Test Statistics (F*), I calculated my Critical Value (F0) for each season to season(s) comparison. To do this, I found the degrees of freedom of each sample and selected a few relevant significance levels. After this, I ran my tests.
I started by testing the pool of 2019-2017 seasons against each individual 2019, 2018, and 2017 season at a .01 significance level (99% Confidence Level) - to ensure that the pool of variances from these seasons could be considered statistically equivalent to the years contained within it. If the Test Statistic (F*) was less than or equal to the Critical Value (F0), then the variances, and thus statistics, could be considered statistically equivalent.
In this experiment, my Null Hypothesis (H0) stated that the 2020 season's variances were equivalent to the pooled 2019-2017 seasons' variances. My Alternative Hypothesis (H1) stated that these two samples were not equivalent to one another. If the Null Hypothesis (H0) was not rejected, a "YES" appeared in the corresponding cell, otherwise, a "NO" appeared in the corresponding cell. Below are the Critical Values (F0) used in my tests as well as the results of each of those tests.


As it turned out, the pool of 2019-2017 seasons could be considered statistically equivalent to each 2019, 2018, and 2017 season according to every statistic at a .01 significance level - signifying that we can be 99% confident that they are equivalent to one another. This gave me the assurance to compare 2020's variances against a much larger, and proven consistent, sample of variances.
With this newfound knowledge, I began testing the 2020 season's variances against the pool of 2019-2017 season variances at lesser and lesser confidence levels. I ultimately ended up testing these samples against seven different levels (99%, 95%, 90%, 87.5%, 85%, 80%, and 75%). Below are the Critical Values (F0) used in my tests, sorted by their level of significance as well as the results of each of those tests. For this series of tests, I utilized the same Null Hypothesis (H0), Alternative Hypothesis (H1), and set of conditional cell outcomes.
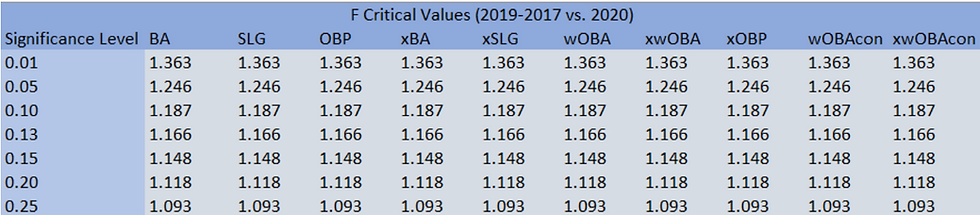
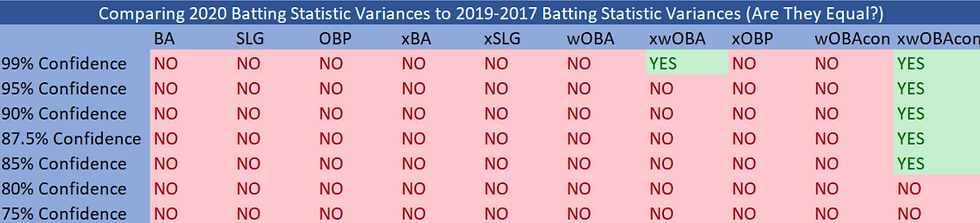
As one might suspect, the variance of nearly all of the most popular statistics used to evaluate hitters from this past season cannot be considered equivalent to previous years. In other words, we should put little to no stock into these statistics for comparison purposes. The only two metrics that actually did prove to be comparable to prior years were xwOBA and xwOBAcon, but we'll talk more about those later on.
Application
This analysis is especially relevant in the case of Wil Myers, veteran outfielder for the San Diego Padres. Myers had a career offensive year this past season, well-exceeding anything he had accomplished in his career coming into 2020.

For almost the entirety of his eight-year career, Myers was a slightly above average hitter in terms of SLG. However, thanks to a severely reduced season length in 2020, he was able to post offensive numbers that far exceeded his career averages, especially in the power department.
In 218 plate appearances this past season, Myers walloped 16 home runs and posted an unsustainably high Home Run To Fly Ball Rate (HR/FB) of 27.8% (the league average was 10.5% in 2020). This performance culminated in a remarkable .606 SLG, which ranked eighth among all qualified hitters in 2020 but represented a .159 increase from his career average. As you may remember from the first graph in this article, SLG possessed one of the largest season-to-season(s) changes in variance, which is why we should put little to no stock into the statistic for the 2020 season.
Despite Myers' increased exit velocities in 2020, the outfielder outperformed all of his expected metrics in a year when many believed he would continue to decline. This analysis suggests that Myer's inflated SLG may be just that. While this is not to say that Myer's 2020 was a complete anomaly, it is safe to say that we should not expect to see the prolific power he put on display this past season again in 2021.
This analysis is also relevant in the case of a player like Mike Yastrzemski, the San Francisco Giants outfielder who posted an impressive .400 wOBA and 160 wRC+ this past season. While both of these statistics identify the sophomore hitter as a breakout star, the expected metrics tell quite a different story.

Looking at the spread between Yastrzemski’s xwOBA (.355) and wOBA (.400) in 2020, it is clear that he underperformed, at least in terms of expected metrics, by a good amount this past season. Upon examining his full slate of expected metrics, the outfielder’s sophomore campaign was actually eerily similar to that of his rookie one, during which he posted a notable, but by no means exceptional, wRC+ of 121.
Yastrzemski has maintained remarkably consistent wxOBAs over his first two seasons in the league, and this analysis identified xwOBA as one of the only reliable statistics from 2020. Thus, it is safe to assume that the 30-year-old outfielder is much more like the hitter we saw in 2019 than the breakout star we witnessed in 2020.
In short, Wil Myers and Mike Yastrzemski both posted impressive 2020 campaigns. However, their expected metrics and the league-wide volatility of other statistics this past season suggest that their performances were not indicative of their true talent level.
Now, onto the two metrics that actually did prove to be comparable to prior seasons: xwOBA and xwOBAcon. Again, since these metrics possessed variances in 2020 that were low enough to be considered statistically equivalent to prior years, we can be more confident when using them for comparison.
However, I should note that because of its lower confidence level threshold, we can be slightly more confident when performing comparisons using xwOBAcon than xwOBA. Intuitively, this makes sense because xwOBAcon relies almost entirely on exit velocity and launch angle - making it more stable than xwOBA, which also factors in strikeouts, walks, and a few other factors.
Despite the increased reliability of xwOBAcon, the more predictive of the two metrics in terms of wOBA is actually xwOBA. For this reason, we should focus more on xwOBA when attempting to predict future offensive performances based on 2020 data. If you would like a more in-depth look at this, I would refer you to this article from the MLB Technology Blog.
Put into practice, this means that a player like Marcell Ozuna, whose .417 xwOBA and .509 xwOBAcon both ranked fourth among qualified hitters this past season had a true comeback season.
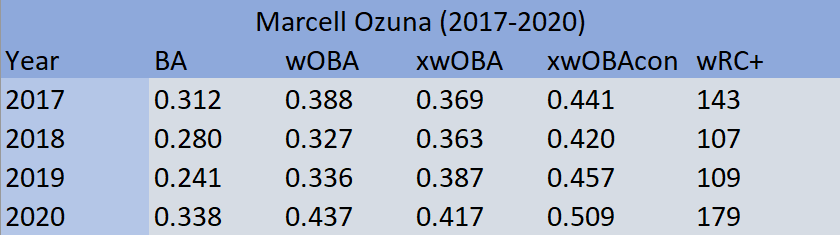
Coming into 2020, the Atlanta Braves outfielder and designated hitter was coming off two underwhelming seasons in St. Louis, where he greatly underperformed his expected metrics. In 2018 and 2019, Ozuna posted spreads between his xwOBA and wOBA of .036 and .051, respectively. This resulted in him posting two pedestrian offensive seasons after a breakout campaign in 2017.
According to wRC+, Ozuna was a slightly above average hitter in each of his two seasons as a Cardinal, which likely resulted in him having to take a one year ‘prove it’ deal with Atlanta. While Ozuna’s resurgence could have been foreseen due to his underperformance in expected statistics, it is still reassuring to see a Silver Slugger caliber player get back on track, especially in his free-agent year.
Thanks to this analysis, we can be confident that despite an impressive performance in a shortened season, Marcell Ozuna is still the elite slugger we saw back in 2017.
This analysis also suggests that someone like Bryce Harper, who most fans regard as a streaky hitter, but maintains consistent year to year xwOBAs and xwOBAcons, may not be as volatile as previously thought.
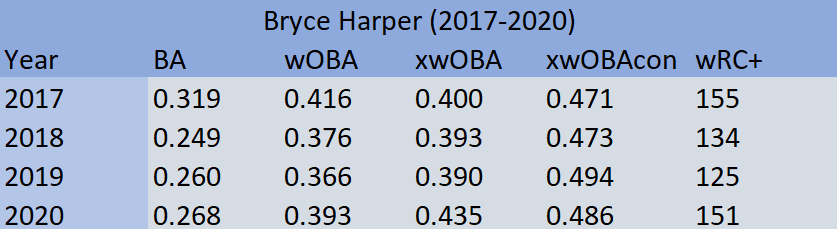
In 2020, the Philadelphia Phillies right fielder posted a career-high .435 xwOBA and a .486 xwOBAcon, which ranked third and eighth among qualified hitters, respectively. While casual fans may give Harper credit for a great 2020 campaign, what they likely do not realize is that over the past four seasons, he has actually been quite consistent.
Between 2017 and 2019, Harper’s xwOBAs remained between .390 and .400, and his xwOBAcons remained between .471 and .494. That combination of consistency and excellence is rarely seen, especially from a hitter who has seen his surface-level statistics, including BA, wOBA, and wRC+, fluctuate as heavily as Harper has in recent seasons. Based on our analysis, the former number-one overall pick should be considered far more consistent than many give him credit for.
In short, Harper and Ozuna both saw declines in their offensive production in 2018 and 2019. However, their xwOBAs (and in Harper’s case, xwOBAcons) remained comparable to those of their peak offensive seasons, suggesting that they did not regress and remained elite hitters.
Conclusion
So, let's close things out by revisiting those two all-important questions we asked ourselves towards the beginning of this article: "Was Major League Baseball's 2020 season, in terms of the variance of its offensive metrics, statistically distinct from those of years past?" and "Which statistics from this past season, if any, had variances similar to that of a full-length season?"
To answer the first question, the answer here is a pretty firm "No." Based on this analysis, a shortened season, and thus a limited sample size, rendered this past season’s offensive metrics statistically distinct from previous ones. Thus, nearly all of the most important statistics used to evaluate hitters from the 2020 season cannot be used as adequate tools for comparison.
To answer the second question, the answer here is a pleasantly surprising "xwOBA and xwOBAcon." Thanks to this analysis, I was able to identify xwOBA and xwOBAcon as the two statistics that had variances low enough that they could be considered comparable to a full-length season. Thus, they can and should both be used for comparison.
Before concluding this article, I should remind you that, like most things, we should examine each player's performance this past season on a case-by-case basis. What may be a wild overperformance for one hitter may just be a random statistical fluctuation for another.
In Wil Myers' case, an uncharacteristically high SLG suggested that his prolific power this past season could have been just a fluke. In Mike Yastrzemski's case, a large spread between his expected metrics and surface-level statistics indicated that he overperformed mightily in 2020. In both situations, a shortened season allowed each of these players to flourish, despite how good of a hitter they truly were.
Meanwhile, in the case of Marcell Ozuna, career-highs in xwOBA and xwOBAcon after years of severe underperformance affirmed that he is still the elite slugger we saw back in 2017. In the case of Bryce Harper, a terrific performance in terms of xwOBA and xwOBAcon in 2020, along with excellent performances according to those metrics in prior years, solidified his superstar status. For both of these players, a shorter season allowed them to affirm their greatness.
It is also important to remember that no one model can perfectly predict future performance, especially in the wild world of sports. While this analysis took into consideration hundreds of qualified hitters in an attempt to identify which statistics from this past season were the most reliable, individual players will certainly challenge the findings of this research.
At the end of the day, it’s always interesting to try to predict how players will perform. And given this past year’s 60 game season, the process of doing so has become more difficult than ever before. Hopefully, this analysis has given you a meaningful insight into which statistics to trust as we look back at 2020 and head into 2021. But as with any set of predictions - we’ll just have to wait and see.
Sources
Comments